关键字 |
GA, TLBO,太阳能、风能 |
介绍 |
能源可再生能源被视为一个可行的替代化石燃料。其中,风能和太阳能的能量已经快,过去十年的重大突破。此外,他们可以在本地消费,因此减少影响从高压输电线路到农村和城市景观和功率损耗。然而,太阳能和风能的独立系统可以完全满足负荷消费,因为季节性和周期性气候变化。因此更可靠和有效的安装一个混合能源系统与存储,因为可再生能源的间歇性字符[1]。全球环境问题和能源不断增长的需求,再加上一个稳步推进可再生绿色能源技术,正在打开新的机遇的利用可再生能源资源。特别是,风力和光伏(PV)生成技术的进步已经增加了他们在最终使用,PV-alone,混合PV-wind配置。此外,这些可再生能源技术是充分的经济方面的承诺目前包括他们的市场的发展[2]。混合能源系统由两个或两个以上的能源系统、储能系统、电力空调设备和控制器。混合能源系统可能是也可能不是连接到电网。他们一般都是大型集中式独立的电网,在农村偏远地区使用[3 - 5]。 In many remote areas of the world, the availability, reliability, and cost of electricity supplies are major issues. The standard solution is typically to use diesel or petrol generators to meet power requirements in areas distant from established electricity grids (Sustainable Energy Development Office 2010). There can be a number of problems with running stand-alone diesel or petrol generation, including noise, pollution, and high running and maintenance costs. Generators can also be inconvenient to use. Due to the high running and maintenance costs, continuous operation of a generator may not be financially viable [6]. The use of hybrid energy systems, incorporating PV and wind resources, in remote locations can overcome or at least limit some of the problems associated withgenerator only systems. The use of these renewable energy-based systems could help reduce the emissions (such as COx, NOx and SOx) [7]. But such PV-wind hybrid systems are usually equipped with diesel generators to meet the peak load demand during the short periods when there is a deficit of available energy to cover the load demand [7, 8]. To eliminate the need of a diesel generator, a battery bank can be used. Battery life is enhanced when batteries are kept at near 100% of their capacity or returned to that state quickly after a partial or deep discharge [8]. The use of PV modules only does not protect batteries against deep discharges. During periods of little or no sunshine, the load draws more energy than the PVs can replace. A more dynamic source of energy is a wind turbine. Adding a wind turbine to a system would protect batteries against deep discharges and thus extend their life [8-10]. Many studies have been carried out in the area of sizing of PV-wind hybrid energy systems. Generally, there are three main approaches to achieve the optimal configurations of such hybrid systems in terms of technical analysis and economic analysis. These approaches are the iteration approach [8-14], the probabilistic approach[15], and the trade-off approach[16]. However, these approaches are time-consuming and difficult to adjust if insolation, wind speed, load demand, rating of each generator, and initial cost of each component are changed. |
文献调查 |
当独立能源系统在光伏板只有或风力涡轮机只与混合PV-wind能源系统相比,混合动力系统更经济可靠的根据气候变化(17、18)。太昂贵的太阳能电池板和权力通过使用他们的生产成本通常高于传统的过程,它不是在夜间或阴天。类似的风力涡轮机不能在高或低风速运行。混合能源系统通常由两个或两个以上的可再生能源一起使用可以提高系统效率以及更大的平衡能源供应。所以这些非线性软计算技术可以解决的问题,但是这对于多目标函数可能并不准确。例如模糊逻辑和神经网络软计算技术,它可能无法提供精确的结果相比,启发式方法,如遗传算法和PSO [13]。 |
混合动力系统结构 |
日益增长的能源需求和环境问题引起了相当大的兴趣在混合可再生能源系统和它的后续发展。代的风能和太阳能是非常依赖于天气条件。因此,没有单一的能源能够提供具有成本效益和可靠的电力。联合使用多个电力资源可以是一个可行的方式来达到平衡的解决方案。结合的可再生系统,功率波动可能会发生。减轻甚至消除波动,能源储存技术,如蓄电池(SBs)可以使用。适当的存储系统是网站特定的大小,取决于可再生发电和负荷的数量。所需的存储容量时可以减少到最低的适当组合使用风能和太阳能发电对于一个给定的网站。提出的结构PV-wind混合能源系统是图1所示。因为大多数电器使用交流电(AC)电源、负载之前使用一个逆变器。为了保证逆变器正常工作,使用转储负载消耗多余的能量由系统生成。 The peak power trackers will keep the PV array and wind generator operating at their maximum power operating points. The proposed method is to optimally size a PV-wind hybrid energy system to electrify a residential remote area household as shown in Fig. 2. |
提出问题的优化方法 |
由于间歇太阳能辐射和风速特点,高度影响产生的能源生产,光伏的主要方面在设计和WG发电系统的可靠电力供应消费者在不同大气条件下和相应的系统总成本。考虑到广泛的商用设备类型,有必要选择光伏模块的数量和类型,WGs和电池,他们的安装细节,权力是不间断地提供给负载,同时最小系统成本。forhybrid PV / WG系统设计方法,提出了基于能量平衡,[19]。使用每小时平均风速数据,太阳辐射和消费者的电力需求,生成并要求权力的差异(Pd)任何时候是24小时为一个周期计算。光伏模块的数量和WGs终于选中时,使用一个迭代过程,系统操作模拟各种数字的PV和WGs这样(Pd)的平均值为零。每个配置的年度总成本计算和成本最低的组合被选中代表最优混合。方法选择最优组合电池和光伏阵列的混合光伏/ WG系统已经由[8]。根据提出的方法[16]的最佳大小的PV和WG电源和电池是由最小化系统总成本函数使用线性规划技术。系统总成本包括初始成本和年度运营和维护成本。上述方法的共同缺点是,提出分级方法不考虑系统设计特点,如电池充电器的数量; the PV modules tilt angle and the WG installation height, which highly affect both, the resulting energy production and the installation and maintenance costs. Also, the minimization of the system cost function has been implemented either by linearly changing the values of the corresponding decision variables or employing linear programming techniques, resulting in suboptimal solutions and increased computational effort requirements.In this paper, an alternative methodology for the optimal sizing of stand-alone PV/WG systems is proposed. The purpose of the proposed methodology is to suggest, among a list of commercially available system devices, the optimal number and type of units ensuring that the 5-year round total system cost is minimized subject to the constraint that the load energy requirements are completely covered, resulting in zero load rejection. The 5-year round total system cost is equal to the sum of the respective components capital and maintenance costs. The decision variables included in the optimization process are the number and type of PV modules, WGs and battery chargers, the PV modules tilt angle, the installation height of the WGs and the battery type and nominal capacity. The minimization of the cost (objective) function is implemented employing a genetic algorithms (GA) approach, which compared to conventional optimization methods, such as TLBO has the ability to attain the global optimum with relative computational simplicity. GAs have been applied to the design of large power distribution systems and the solution of power economic dispatch problems because of their ability to handle complex problems with linear or non-linear cost functions both, accurately and efficiently. In the proposed method, GA is selected because it has shown to be highly applicable to cases of large nonlinear systems, where the location of the global optimum is a difficult task. Due to the probabilistic development of solutions, GA is not restricted by local optima. Thus, given the number of non-linearities that exist in similar problems; GA appears to be a useful approach. A general block diagram outlining the proposed methodology is shown in Fig. 3. |
气象和加载数据 |
该方法是最优规模PV-wind混合能源系统使住宅为偏远地区家庭附近纬度39.74˚N,经度105.18˚W,时区:GMT-7,海拔高度:-1829米。MIDC / NREL太阳辐射研究实验室(BMS)是一个很好的来源的长期月度平均每日太阳辐射数据(事件水平和朝南光伏阵列由站点的纬度角ϕ倾斜)和风速数据(以42英尺/ 12.8米高度的网站)。该方法需要一个长期风速数据记录和全球日晒数据(事件朝南光伏阵列由网站纬度角ϕ倾斜)每个月的每一天都在一段时间的1年。图4和图5显示这些数据(即。,the global solar insolation and windSpeed, respectively) for every month in a typical year. Fig.6 illustrates the considered residential remote area load profile, during the 12 months of the year. |
问题公式化 |
的设计中主要关心的问题提出PV-wind混合能源系统是确定每个组件的大小参与系统,负载能够可靠地在经济和满足。因此,系统组件发现主题:1。降低系统的总成本(CT), 2。确保负载按照一定的可靠性标准。目标函数(CT)最小化,这代价函数生成的现值的总和(PWs)的救助值的设备、年度运营和维护成本,初始投资或资本,系统组件的更换成本。因此,目标函数可以被制定为: |
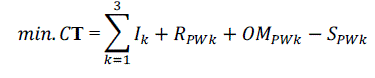 |
指数k是占光伏、风能、和电池;本土知识是每个组件的资本或初始投资k;RPWk PW的每个组件k的重置成本;OMPWk的PW k每个组件的操作和维护成本;SPWk是每个组件的残值的PW k。应该满足的约束,同时最小化目标函数CT,应该确保负载按照一定的可靠性提供标准。 |
答:基本经济考虑 |
方程(1)表明,一些年度支付的规模以及救助的价值观是必要的。因此,假设为项目N年的生活视野,r,利率和通货膨胀率j(价格上涨所致),可以计算不同规模如下[20]。 |
1)残值 |
如果一个组件的残值年代(Rs)目前(因为它将结束其总或生命周期),那么组件的残值将S (1 + j) N(即。,N年后提供组件放在服务目前)。S的PW (1 + j) N,考虑到利率[20]: |
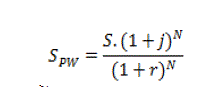 |
让fac1 = (1 + j) N / (l + r) N,然后SPWk = Sk facl,混合动力系统的所有组件k。 |
2)操作和维护 |
如果一个组件的操作和维护成本OM (Rs /年),那么这个倾向于每年升级速度不一定等于通货膨胀率。因此,对于升级每年es的操作和维护费用将OM (1 + es) y,和PW的: |
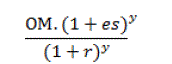 |
PWs的年度支付的总和,因此,由: |
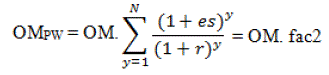 |
fac2代表一个几何级数,是由: |
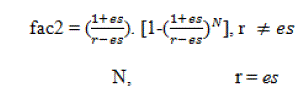 |
因此,OMPWk = OMk。fac2为系统中所有的组件k。注意,其他PW计算将以类似的方式在每个组件的分析。 |
b .总成本系数 |
1)光伏阵列 |
假设设计变量,在光伏阵列,阵列总面积APV平方米。这个区域都受到光伏阵列的最大可用面积(例如建筑的屋顶表面)和thebudgetpresetfor PV模块。的初始成本αPV (Rs / m2),初始投资总额是: |
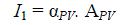 |
这里请注意,如果项目寿命被认为是一样的光伏阵列,那么光伏模块的重置成本将是微不足道的(也就是说,RPW1 = 0)。的年度运营和维护成本αOMPV (Rs /平方米/年),总年度运营和维护成本将OM1 =αOMPV。APV。因此,每年的全球PW操作和维护成本将是: |
 |
可以找到残值乘以每平方米售价APV SPV的区域,和PW的销售价格是: |
 |
总之,光伏阵列的规模成本: |
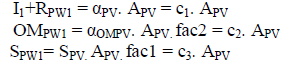 |
2)风力涡轮机 |
的设计变量由于使用风力发电机的转子横扫总面积Aw平方米。这个值是受制于可用空间和项目的预算。注意,如果Aw是已知的,那么它是设计师的任务分发Aw在几个机器这样的总和个人领域给了啊。由于风力涡轮机Lw通常是短的生命周期比PVarray N,那么它可能需要购买额外的风力涡轮机之前项目的寿命结束。的次数,withinNyears风力涡轮机需要Xw = N / Lw(圆大的整数)。如果αw价格目前在Rs。/ m2,今年价格ywouldαw。(1 + es) y的PWαw。(1 + es) y y / (1 + r)。因此,所有初始和替换的PW投资风力涡轮机是[20]: |
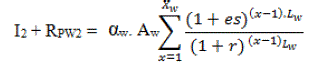 |
es升级速度,r是利率,Lw是风力发电机的寿命,Xw风力涡轮机是购买的次数。注意,如果Xw = 1(即。,the life span of the wind turbines is greater than or equal to that of the whole project), then RPW2 = 0 and I2 = αw.Aw (since the wind turbines are bought once at the beginning of the project). With a yearly operation and maintenance cost of αOMw (Rs. /m2/year), the total yearly operation and maintenance cost would be OM2=αOMw.Aw, and the PW of all the yearly costs would be: |
 |
残值的假定风力涡轮机降低线性αw (Rs / m2) Sw (Rs / m2),当风力发电机运行在其一生Lw(即。,从安装到它的生命周期,分别)。如果项目生命结束前的风力涡轮机已经达到其寿命的结束,然后在SPW风力涡轮机可以出售(Rs / m2),这是一个价值大于西南。 |
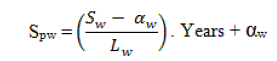 |
“年”表示操作的数年之间最后一个风力涡轮机的安装和项目生命周期的结束。因此,找到所有打捞值的PW: |
 |
如果N(即。,the life span of the project) is a multiple of that of the wind turbines Lw, then Equation (12) can be reduced to: |
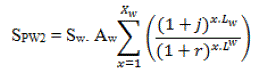 |
总之,风力涡轮机的规模: |
 |
 |
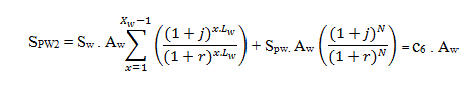 |
3)存储电池 |
设计变量在蓄电池容量Cb千瓦时。在风力发电机的情况下,电池的寿命磅预计将低于整个项目。因此,电池容量的Cb定期购买的磅。总PW的资本和更换电池是由投资: |
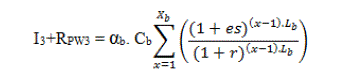 |
磅的电池寿命,Xb是电池的次数应该购买项目生命周期期间,Xb = N /磅(圆形大整数),andαb的资本成本(Rs /千瓦时)。残值的电池被认为是微不足道的。的年度运营和维护成本αOMb (Rs /千瓦时/年),总年度运营和维护成本将OM3 =αOMb。Cb和PW的年度成本是: |
 |
总之,电池成本的规模: |
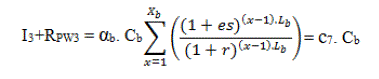 |
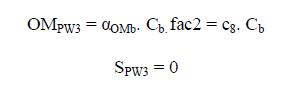 |
c .系统建模 |
建模是一个重要的步骤在任何阶段的最优规模。提出了PV -风与蓄电池混合系统,如图1所示,包括三个主要子系统,光伏阵列,风力涡轮发电机(WTG),和电池存储。 |
1)光伏阵列的造型 |
在该方法中,系统操作是模拟一年时间步的1 h。光伏能源产生的电力是假定为常数在那段时期。因此,可再生能源发电的数值相等的能量在这个时间步生成的。光伏阵列产生效率ηPV和区域APV (m2), PPV输出功率(千瓦),当受到可用的太阳能日晒R(千瓦/ m2)倾斜的表面上,是由[12]。 |
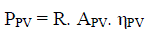 |
在这里,光伏阵列上的日晒R事件定义如图4所示。 |
2)WTG的造型 |
WTG产生的电量Pw高于切入风速V时速度Vci和V是高于时关闭断路器Vco速度。当虚拟现实< V < Vco (Vr额定风速),WTG产生额定功率公关。如果Vci < V < Vr, WTG输出功率变化根据多维数据集。使用下列方程为模型WTG [8、14]: |
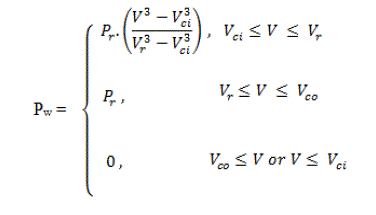 |
在哪里 |
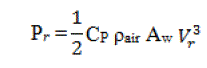 |
在上面的方程中,Cp、ρair和Aw功率系数,空气密度,分别和转子扫掠面积。可用的风速数据Vi(见图5)估计高度嗨= 12.8米,然后这些数据升级到一个特定的中心高度H,下列方程常用的[2,8]: |
 |
V是升级后的风速在中心高度H和幂律指数(1/7≈开放土地)。 |
3)蓄电池的建模 |
在任何时刻t,电池的电荷状态(SOC (t))与前面的电荷状态(SOC (t - 1))和系统的能源生产和消费情况在时间t 1 t。在充电过程中,当电池PB流向电池(即。,PB > 0),可用电池电荷状态在时间t可以被描述为: |
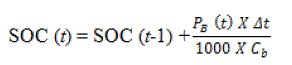 |
Δt哪里仿真时间步(这将等于1小时),和Cb总电池的额定容量千瓦时。另一方面,当电池供电,电池外(即流动。PB < 0),电池处于放电状态。因此,可用电池电荷状态在时间t可以表示为: |
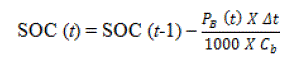 |
延长电池寿命,电池不应过量放电或充电。这意味着电池SOC在任何时刻t必须服从以下约束: |
 |
在DODmaxand SOCmax电池最大容许放电深度和电荷状态,分别。 |
系统可靠性和模拟 |
首先,它假设,在这工作,峰值功率跟踪器,电池充电器/放电器,是理想的(即分布线。,他们是无损的)。同时,假设逆变器效率ηinv是常数,电池充电效率ηb设置为等于制造商的往返效率,电池放电效率将是1。产生的总功率的光伏阵列和WTG小时t, Pg (t)可以表示为: |
 |
是指出所需的负载需求在任何时刻t, PL * (t) Pg (t)可能是也可能不是满足根据相应的值产生的总功率Pg (t)和可用电池SOC (t)小时。PV-wind混合动力系统的提出能源管理可以概括如下: |
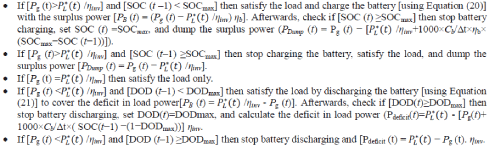 |
假设,在这部作品中,仿真时间步Δt = 1 h和生成的光伏和风能大国在Δt是常数。然后,在Δt功率数值等于能量。程序的计算建议PV-wind混合系统是用MATLAB写的代码在一个m文件。这个程序的流程图图是图9所示。这个程序的输入数据包括平均每小时全球一系列倾斜日晒R,平均每小时风速Vi和所需的负载功率年内����。这里要注意,每提出PV-wind混合动力系统的配置,这个程序模拟系统。有三个额外的范围,应该对系统组件的大小,这是: |
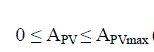 |
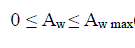 |
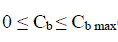 |
最终形式和优化技术 |
在这个阶段,可以编写优化问题的最终形式如下: |
•最小化代价函数CT |
 |
•主题: |
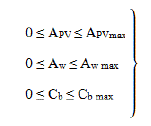 |
解决上述优化问题,提出了软计算方法(GA),在那里,在这个工作[21],遗传算法在MATLAB软件代码用于解决前面的优化问题。GA包含精英方法[22]。这意味着一个解决方案不能从一代一代的降解,但这一代最好的个人复制到下一代没有做任何更改。使用遗传算法,求解优化问题,一个m文件(MATLAB代码)写了,计算目标函数的值(或称为适应度函数)。编写的m文件必须接受一个向量(即。,individual) whose length is the number of independent variables for the objective function and return the corresponding scalar values of the objective function (i.e., cost).In this work, the individual of the considered optimization problem contains three variables (or genes), which are: APV, Aw, and Cb.The used GA is based uponusing the flowchart of Fig. 8, to yield the optimal solution. Initially, the GA selects individuals at random from the current population to be parents and uses them to produce the children for the next generation by using the three main operations, which are the selection, crossover, and mutation operations. Then, it can repeatedly modify a population of individual solutions, where, over successive generations, the population evolves toward an optimal solution. Note, here, that the used different settings in the GA are 100 individuals for the population size,the stochastic uniform function for the selection operation, the scattered crossover function (with a crossover probability of 80%) for the crossover operation, the adaptive feasible mutation function (with a probability rate of 1%) for the mutation operation, and an elite individual. At the same time, it is to be noted that the additional three bounds of Equation (28) can be entered directly in the dedicated positions of the GA. |
一个名叫基于教学学习最新的优化算法,优化(只是TLBO)提出了r . v . Rao et al,在2011年,用于处理机械的优化设计问题,考虑到教师对学习者的影响。之后,进行的一些改进和实际应用对TLBO算法。然而,据我们所知,没有这样的作品分类当前工作TLBO从代数和分析的观点[26]。该算法模拟老师的教学能力和学习者在课堂上。教师和学生是两个算法的重要组件。算法描述学习的两种基本模式:(1)通过老师(称为老师阶段)和(2)与其他学习者交互(即学习者阶段)。TLBO算法的输出是的结果或学习者的成绩,这取决于教师的质量。高质量的教师通常被认为是高度学习训练学习者的人,这样他们就可以实现更好的结果的标志或者成绩。此外,学习者还通过互动学习,这也有助于提高他们的结果。r . v . Rao等人证明TLBO可以在许多领域获得更好的优化性能,例如,受约束的机械优化设计问题,无约束和实参的约束优化问题,大规模和连续非线性问题,与其它优化算法相比。然后,许多改进和应用提出了关于TLBO顺序[26]。The basic procedure for implementing the TLBO algorithm is as follows:Initialize the population size, design variables and termination criterion, evaluate the initial population, Calculate the mean of each design variable and select the best solution, Calculate the difference mean (Difference*, D = r (Mnew, D- TF X M*, D) and modify the solutions based on best solution, The existing solution is updated as in Xnew, D = Xold, D + Difference*, D, If new solution is better than existing then select the solutions randomly and modify them by comparing with each other and if not than keep the previous solution and repeat step 3-5, If termination criterion is fulfilled then take the final value of the solution. Fig 9 shows the flow chart of TLBO algorithm and its operation for non-linear problems. |
应用结果与讨论 |
PV-wind混合能源系统的制定优化问题已经解决了,在这个工作中,通过使用遗传算法和TLBO在MATLAB软件,可能提供一个给定的问题可能的解决方案。最后的选择最佳的解决方案是系统的设计师。规范和相关维护和安装成本不同的风力涡轮机、太阳能电池板和电池,这是最佳的分级过程的输入,列出表》。每个单元的维护成本和安装成本每年的每个组件分别设定为0 - 1%和5 - 10%相应的成本。风力发电机的寿命,光伏板和电池被认为是5年。风力发电机塔高度以来的结果产生显著影响,在海拔12.8米高的塔选择1829。系统总成本的最小化是通过选择一个适当的系统配置。表4中,它表明不同组件的最佳大小的比较结果包含在混合动力系统中。(即相应的适应度函数优化。,minimization of the system cost in rupees) along the successive generations of the GA is shown in Fig 10, which indicates that the system is optimized after forty iterations only. Similarly In another M-file a MATLAB code has written for proposed hybrid PV/Wind energy system using Teaching Learning Based Optimization (TLBO) algorithm. The corresponding fitness function optimization (i.e., minimization of the system cost in rupees) along the successive iterations of the TLBO is shown in Fig 11, which indicates that the system is not optimized even after hundred iterations. Table-VI shows a comparison between the costs that resulted from solving the formulated optimization problem by using the proposed GA-based technique and the TLBO algorithm using MATLAB software. Thus, Table-V indicates that the proposed GA-based technique is better than the TLBO algorithm, in solving such optimization problems, and this is due to the fact that the GA is capable to converge to the global optimum solution instead of convergent at a local optimum one. Fig 12 shows the comparison of result using GA and TLBO, where GA is most efficient than other.Figure 13, 14, and 15 illustrates the generated PV power, wind power, and the total generated power of the suggested PV-wind hybrid system, for every month during the year. |
结论 |
本文提出了ga TLBO-based优化技术优化大小提出PV-wind混合能源系统,包含一个存储电池。优化问题是制定,在这工作,达到最低总成本的系统组件,并确保负载提供可靠。GA收敛的结果产生非常好,该技术是可行的分级的PV-wind混合能源系统,独立光伏系统,或独立的风系统。此外,该techniquesare能够调整如果日晒,风速、负荷需求,每个组件参与系统的初始成本发生了变化。结果产量,同时,PV-wind混合能源系统是最经济和可靠的解决方案的偏远地区负荷。 |
表乍一看 |
|
数据乍一看 |
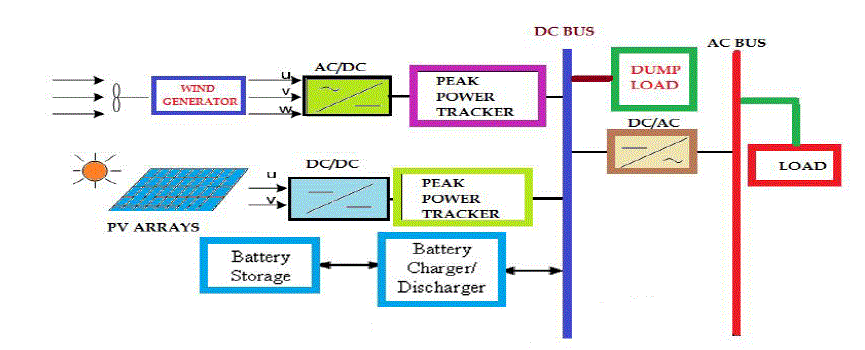 |
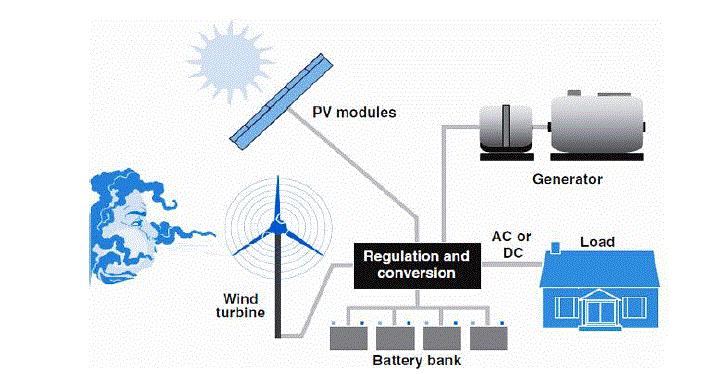 |
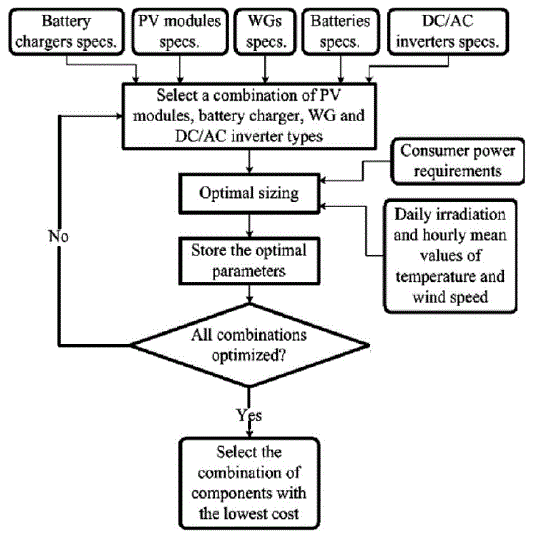 |
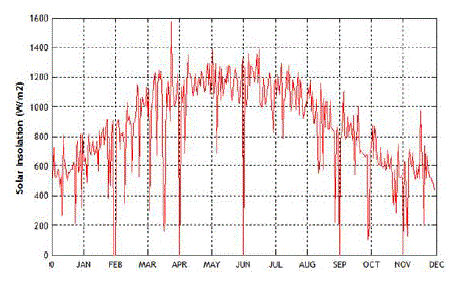 |
 |
图1 |
图2 |
图3 |
图4 |
图5 |
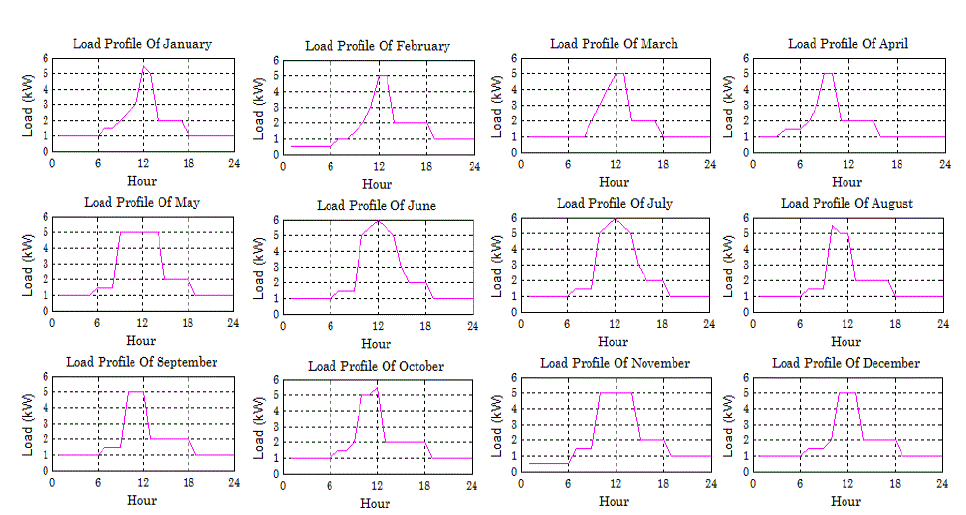 |
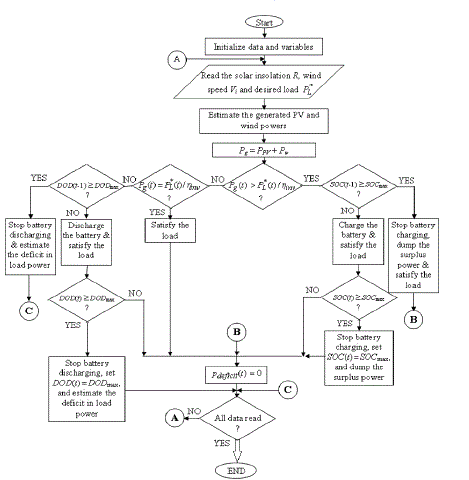 |
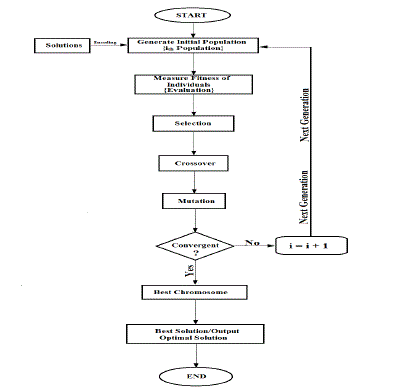 |
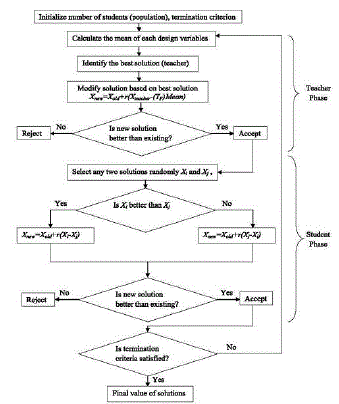 |
 |
图6 |
图7 |
图8 |
图9 |
图10 |
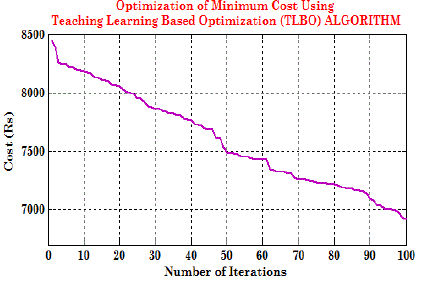 |
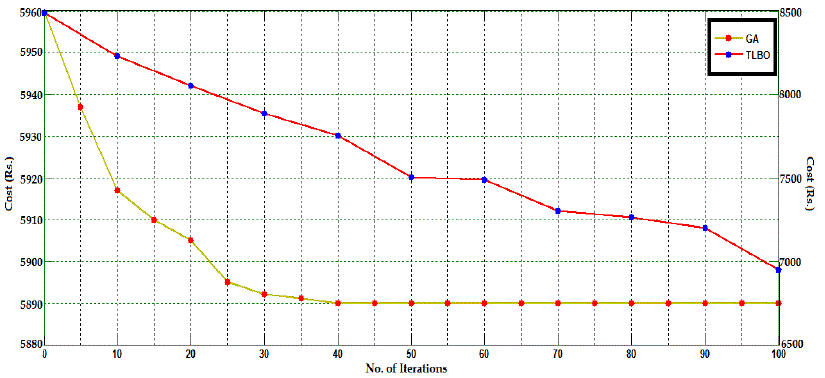 |
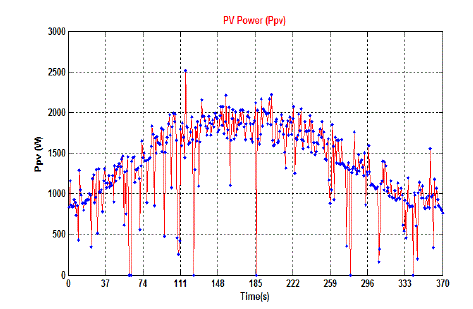 |
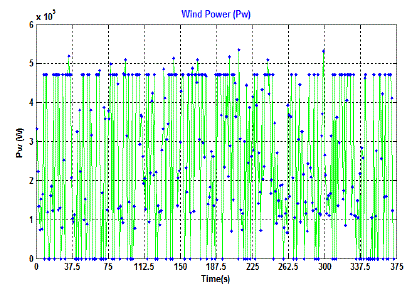 |
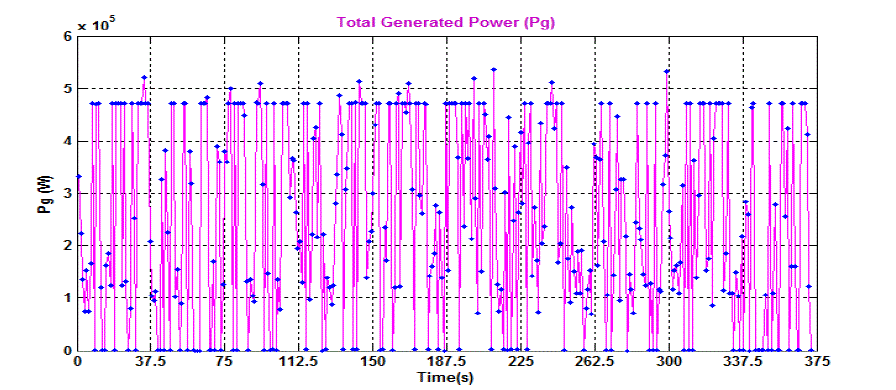 |
图11 |
图12 |
图13 |
图14 |
图15 |
|
引用 |
- .Bernal-Agustin J.L.Dufo-Lopez, R。:“独立混合可再生能源系统的仿真与优化,更新。维持。EnergyRev(8),2009年,13日,页。2111 - 2118。
- 。凯洛格,W.D.,M.H. Nehrir, G. Venkataramanan, and V. Gerez. 1998. Generation unit sizing and cost analysis for stand-alone wind, photovoltaic, and hybrid wind/PV systems.IEEE反式。在能量转换(1):13页:70 - 75。
- 。古普塔,。,R.P. Saini, and M.P. Sharma. 2007. Design of an optimal hybrid energy system model for remote rural area power generation. In电气工程学报IEEE国际会议(2007年ICEE)拉合尔,巴基斯坦,1 - 6。
- 。红星,Y。,Z. Wei, and L. Chengzhi. 2009. Optimal design and techno-economic analysis of a hybrid solar–wind power generation system.应用能源86:163 - 169。
- 。罗马,E。,R. Alonso, P. Ibanez, S. Elorduizapatarietxe, and D. Goitia. 2006. Intelligent PV module for grid-connected PV systems.IEEETransactions工业电子产品53 (4):1066 - 1073。
- 。穿过公路,S。,S. Luna, and R. Ricardo. 2004. Economic evaluation and optimization of hybrid diesel/PV systems integrated to utility grids.SolarEnergy76:295 - 299。
- 。葡萄酒,前作空。,R.A. Johnson, A.N. Agrawal, and T.J. Chubb. 2005. Simulink model for economic analysis and environmental impacts of a PV withdiesel-battery system for remote villages.IEEE电力系统20 (2):692 - 700。
- 。Borowy,狗屁,and Z.M. Salameh. 1996. Methodology for optimally sizing the combination of a battery bank and PV array in a wind/PV hybridsystem.IEEE能量转换11 (2):367 - 375。
- 。普拉萨德,境,and E. Natarajan. 2006. Optimization of integrated photovoltaic-wind power systems with battery storage.能源31日:1943 - 1954。
- 。Shaahid,克里,and M.A. Elhadidy. 2008. Economic analysis of hybrid photovoltaic-diesel-battery power systems for residential loads in hotregions-a step to clean future.可再生能源和可持续能源的评论雷竞技苹果下载12:488 - 503。
- 。Ai, B。,H. Yang, H. Shen, and X. Liao. 2003. Computer aided design of PV/wind hybrid system.可再生能源28日:1491 - 1512。
- 。Diaf, S。,D. Diaf, M. Haddadi, and A. Louche. 2007. A methodology for optimal sizing of autonomous hybrid PV/Wind system.能源政策35:5708 - 5718。
- 。Senjyu, T。,D. Hayashi, N. Urasaki, and T. Funabashi. 2006. Optimum configuration for renewable generating systems in residence usinggenetic algorithm.IEEE能量转换21 (2):459 - 466。
- 。杨,H。,L. Lu, and W. Zhou. 2007. A novel optimization sizing model for hybrid solar-wind power generation system.太阳能81:76 - 84。
- 。卡拉奇,萨达姆政权,R.B. Chedid, and R. Ramadan. 1999. Probabilistic performance assessment of autonomous solar-wind energy conversion systems.IEEE能量转换14 (3):766 - 772。
- 。Chedid, R。,H. Akiki, and S. Rahman. 1998. A decision support tech. for the design of hybrid PV/wind systems.IEEE EnergyConversion13:76 - 82。
- 。人工智能垃圾桶,杨红星、申恢辽刘晓波,“计算机辅助设计光伏/风力混合系统,”进行3 td WorldConference光伏能量转换,3卷,pp.2411 - 2414, 2003年5月。
- 。Belfkira R,哈吉O, Nichita Barakat C和G,“独立优化分级混合风力/光伏系统与电池存储,”2007年EuropeanConference电力电子和应用程序pp.1-10, 9月,2007年。
- 。http://lcm.csa.iisc.ernet.in/TDM/hybrid.html。
- 。Sweelem,电子艺界,and A.A. Nafeh. 2006. A linear programming technique for optimally sizing a PV-diesel-Battery hybrid energy system.爱资哈尔大学工程杂志9 (3):705 - 716。
- 。Renshburg, P.J.V.,I.S. Shaw, and J.D.V. Wyk. 1998. Adaptive PID-control using a genetic algorithm. In第二届InternationalConference知识智能电子系统,133 - 138。
- 。http://en.wikipedia.org/wiki/Genetic_algorithm。
- 。http://www.civil.iitb.ac.in/tvm/2701_dga/2701-ga-notes/gadoc/gadoc.html。
- 。http://www.doc.ic.ac.uk/ nd surprise_96 /杂志/ vol1 /高分子量/ article1.html #介绍。
- 。http://c2.com/cgi/wiki?GeneticAlgorithm。
- 。饶,随机变数,Savsani, V.J., and Vakharia, D.P., 2011. Teaching–learning-based optimization: a novel method for constrained mechanical designoptimization problems.计算机辅助设计,43岁,303 - 315。
- 。饶,随机变数,Savsani, V.J., and Vakharia, D.P., 2012. Teaching–learning-based optimization: an optimization method for continuous non-linearlarge scale problems.信息科学1 - 15,183。
|